AI Pioneers Unveil Six Major Innovations Shaping 2023: A Milestone in Artificial Intelligence Advancements
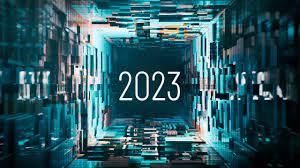
Artificial Intelligence (AI) refers to the simulation of human intelligence in machines that are programmed to think and learn like humans. The goal of AI is to create systems that can perform tasks that typically require human intelligence. These tasks include learning, reasoning, problem-solving, perception, language understanding, and decision-making.
Key components and concepts associated with AI include:
- Machine Learning (ML): This is a subset of AI that focuses on developing algorithms and statistical models that allow computers to perform tasks without being explicitly programmed. Machine learning systems can learn from data and improve their performance over time.
- Deep Learning: Deep learning is a subfield of machine learning that involves neural networks with many layers (deep neural networks). It has been particularly successful in tasks such as image and speech recognition. Deep learning models attempt to simulate the way the human brain works, allowing them to automatically learn and make decisions from data.
- Natural Language Processing (NLP): NLP is a branch of AI that enables machines to understand, interpret, and generate human language in a way that is both valuable and meaningful. Applications include language translation, chatbots, and sentiment analysis.
- Computer Vision: This involves teaching machines to interpret and make decisions based on visual data, such as images or videos. Computer vision has applications in facial recognition, object detection, and autonomous vehicles.
- Robotics: AI plays a significant role in robotics by enabling machines to perceive their environment, make decisions, and execute tasks. This is crucial for tasks in manufacturing, healthcare, and exploration.
- Expert Systems: These are AI systems that emulate the decision-making ability of a human expert in a specific domain. They use knowledge bases of human expertise and an inference engine to solve specific problems.
- AI Ethics: As AI systems become more integrated into daily life, there is a growing emphasis on addressing ethical considerations, including bias in algorithms, privacy concerns, and the impact of AI on employment.
Artificial Intelligence can be categorized into two types:
- Narrow AI (Weak AI): This refers to AI systems that are designed and trained for a particular task. They excel in that specific area but lack the general cognitive abilities of humans.
- General AI (Strong AI): This refers to a form of AI that possesses the ability to understand, learn, and apply knowledge across a broad range of tasks, similar to human intelligence. General AI remains a theoretical concept and is not yet realized.
AI is a dynamic and rapidly evolving field with significant implications for various industries and aspects of society. Researchers and engineers continually work on advancing AI technologies to enhance their capabilities and address challenges.
- Advanced Natural Language Processing (NLP): Continued progress in natural language processing could lead to more sophisticated language models, enabling AI systems to better understand and generate human-like text. This could have applications in chatbots, language translation, and content creation.
- Explainable AI (XAI): As AI systems become more complex and integrated into critical decision-making processes, the need for explainability and transparency grows. Innovations in XAI aim to make AI algorithms more understandable and interpretable for humans, especially in fields like healthcare and finance.
- AI in Healthcare: Enhanced applications of AI in healthcare, including diagnostic tools, personalized medicine, and drug discovery. AI could contribute to more efficient and accurate disease detection, treatment planning, and medical research.
- AI for Cybersecurity: The use of AI in cybersecurity is expected to evolve, with AI algorithms becoming more adept at identifying and mitigating cyber threats. Improved threat detection and response systems may help organizations stay ahead of increasingly sophisticated cyber attacks.
- Edge AI: With the growing prevalence of Internet of Things (IoT) devices, there’s a trend toward pushing AI processing to the edge, closer to the data source. This can lead to faster decision-making, reduced latency, and improved efficiency in various applications, from smart homes to industrial settings.
- AI Ethics and Bias Mitigation: As AI systems play a larger role in decision-making processes, addressing ethical concerns and mitigating biases becomes crucial. Innovations in AI ethics may include the development of tools and frameworks to ensure fair and responsible AI applications.
Remember, these are speculative trends, and the actual innovations in 2023 may vary. To get the most accurate and up-to-date information, refer to recent publications and reports from reputable sources in the field of artificial intelligence.
ChatGPT and other generative AI
ChatGPT is an example of a generative AI model developed by OpenAI. Generative AI refers to models that can generate new content, such as text, images, or other forms of data. These models are often based on deep learning techniques, with neural networks being a common architecture.
Here are some key points about ChatGPT and generative AI:
- Generative Pre-trained Transformer (GPT): GPT is a type of generative AI model that uses a transformer architecture. Transformers have proven to be highly effective in capturing long-range dependencies in data, making them suitable for generating coherent and contextually relevant content.
- Pre-training and Fine-tuning: Models like ChatGPT are typically pre-trained on a large corpus of diverse data to learn general language patterns. After pre-training, the model can be fine-tuned on specific tasks or domains to make it more specialized.
- ChatGPT: ChatGPT is specifically designed for natural language understanding and generation. It is a descendant of models like GPT-3 (the third version of the Generative Pre-trained Transformer). ChatGPT can be used for various conversational tasks, including answering questions, providing information, and engaging in dialogue.
- Interactive Conversations: Unlike traditional chatbots that may follow a rule-based approach, generative models like ChatGPT generate responses based on patterns learned during training. This allows for more dynamic and context-aware interactions.
- Use Cases: Generative AI has found applications in diverse areas, such as content creation, chatbots, language translation, summarization, and even creative writing. The ability to generate human-like text has made generative AI models versatile in solving various natural language processing tasks.
- Ethical Considerations: The deployment of generative AI models has raised ethical concerns, including issues related to bias, misinformation, and the potential for malicious use. Researchers and developers are actively working on addressing these concerns and improving the responsible use of AI.
- Advancements and Research: The field of generative AI is rapidly evolving, with ongoing research aimed at improving model performance, reducing biases, and enhancing interpretability. Researchers are also exploring ways to make models more efficient and environmentally friendly.
It’s important to note that while generative AI models like ChatGPT showcase impressive capabilities, they also have limitations and may generate outputs that are contextually incorrect or biased. Continuous research and development aim to improve the strengths and address the weaknesses of such models for broader and safer applications.
Disease detection through retinal images
Disease detection through retinal images, known as retinal imaging or retinal diagnostics, is an area of medical imaging that has gained prominence due to its non-invasive nature and its potential in early detection and monitoring of various diseases. The retina, being a part of the eye that is sensitive to light, provides valuable information about the overall health of the eye and can offer insights into systemic diseases. Here are some key aspects related to disease detection through retinal images:
- Diabetic Retinopathy (DR) Detection:
- One of the common applications of retinal imaging is in the detection of diabetic retinopathy, a complication of diabetes that affects the blood vessels in the retina.
- Retinal images can reveal changes in blood vessel structure, hemorrhages, and other signs indicative of diabetic retinopathy.
- Age-Related Macular Degeneration (AMD):
- AMD is a degenerative condition affecting the central part of the retina (macula). Retinal imaging can help in identifying the characteristic changes associated with AMD, such as drusen or pigmentary changes.
- Glaucoma Detection:
- Retinal imaging can be used to assess the optic nerve head and retinal nerve fiber layer, aiding in the early detection of glaucoma, an eye condition characterized by damage to the optic nerve.
- Hypertensive Retinopathy:
- Hypertension can affect the blood vessels in the retina, leading to hypertensive retinopathy. Retinal images can show signs such as arteriolar narrowing, hemorrhages, and exudates.
- Retinopathy of Prematurity (ROP):
- ROP affects premature infants and can lead to abnormal blood vessel development in the retina. Retinal imaging is crucial for monitoring and early intervention in these cases.
- Optical Coherence Tomography (OCT):
- OCT is a specialized imaging technique that provides high-resolution, cross-sectional images of the retina. It is valuable for assessing retinal thickness and identifying abnormalities in different retinal layers.
- Artificial Intelligence (AI) in Retinal Disease Detection:
- AI and machine learning algorithms are increasingly being applied to retinal images for automated disease detection.
- These algorithms can analyze large datasets, identify patterns, and assist healthcare professionals in diagnosing and monitoring retinal diseases.
- Telemedicine and Remote Monitoring:
- Retinal imaging, especially when coupled with telemedicine, allows for remote monitoring of patients with retinal conditions.
- This is particularly useful in situations where access to specialized eye care may be limited.
Early detection of retinal abnormalities through imaging can be crucial for timely intervention and better management of various eye and systemic diseases. It is essential to integrate advancements in medical imaging technologies and artificial intelligence to enhance the accuracy and efficiency of disease detection using retinal images. Regular eye screenings and collaboration between ophthalmologists, optometrists, and technology developers are key to advancing this field.
Improvements to medical productivity
Improvements to medical productivity involve enhancing efficiency, accuracy, and effectiveness in healthcare processes to ultimately provide better patient care. Here are several ways in which medical productivity can be improved:
- Health Information Technology (HIT):
- Electronic Health Records (EHRs): Implementing robust EHR systems helps streamline patient information management, reducing paperwork and improving access to crucial medical data.
- Telehealth and Telemedicine: Virtual consultations and remote monitoring technologies can reduce the need for in-person visits, improving accessibility and saving time for both healthcare providers and patients.
- Artificial Intelligence (AI) and Machine Learning (ML):
- Diagnostic Support: AI can assist healthcare professionals in diagnosing diseases more quickly and accurately by analyzing medical imaging, pathology slides, and other diagnostic data.
- Predictive Analytics: Using machine learning algorithms to analyze patient data can help predict disease risks, anticipate patient needs, and optimize resource allocation.
- Workflow Optimization:
- Process Automation: Implementing automation for routine administrative tasks, appointment scheduling, and billing can free up time for healthcare providers to focus on patient care.
- Task Delegation: Efficient delegation of tasks within healthcare teams can ensure that each member operates at the top of their license, maximizing productivity.
- Patient Engagement Solutions:
- Mobile Apps and Patient Portals: Providing patients with tools to schedule appointments, access medical records, and communicate with healthcare providers can enhance engagement and reduce administrative burdens.
- Remote Patient Monitoring: IoT devices and wearables can enable continuous monitoring of patients with chronic conditions, reducing hospital readmissions and improving overall care.
- Interoperability:
- Data Exchange Standards: Improving interoperability among different healthcare systems and devices allows for seamless sharing of patient information, reducing redundancies and enhancing collaboration.
- Health Information Exchange (HIE): Facilitating the exchange of health information among different healthcare organizations improves continuity of care and reduces duplicate tests and procedures.
- Training and Skill Development:
- Continuing Education: Providing ongoing training and professional development opportunities for healthcare staff ensures they stay current with the latest medical advancements and technologies.
- Cross-Training: Cross-training healthcare professionals in various specialties can enhance flexibility in staffing and improve response times.
- Lean Management Principles:
- Reducing Waste: Applying lean principles to healthcare operations helps identify and eliminate unnecessary steps, delays, and resource wastage, leading to more efficient workflows.
- Continuous Improvement: Encouraging a culture of continuous improvement allows healthcare organizations to adapt to changing circumstances and find innovative solutions to challenges.
- Resource Optimization:
- Capacity Planning: Using data analytics to forecast patient volumes and demand for services helps healthcare organizations optimize resource allocation and avoid bottlenecks.
- Supply Chain Management: Efficient management of medical supplies and equipment helps prevent shortages, reduce costs, and ensure a steady workflow.
By embracing technology, optimizing workflows, and fostering a culture of continuous improvement, healthcare providers can enhance their productivity, ultimately leading to better patient outcomes and experiences. It’s important to implement these improvements thoughtfully and with a focus on maintaining high standards of patient care and safety.
Medical imaging and education
Medical imaging plays a crucial role in medical education, offering students valuable insights into anatomy, pathology, and clinical procedures. Here are several ways in which medical imaging is integrated into medical education:
- Anatomy Education:
- 3D Visualization: Medical imaging, such as computed tomography (CT) and magnetic resonance imaging (MRI), provides three-dimensional visualizations of the human body. This aids in a deeper understanding of anatomy compared to traditional two-dimensional illustrations.
- Virtual Dissection: Virtual dissection tools allow students to explore anatomical structures in a non-invasive manner, enhancing their understanding of the spatial relationships between different organs and tissues.
- Clinical Training:
- Simulation: Medical imaging is used in simulation environments to replicate real-life clinical scenarios. Simulated radiographic images, CT scans, and MRIs help students practice interpreting results and making clinical decisions.
- Procedure Planning: Medical imaging is crucial in planning and simulating various medical procedures, such as surgeries and interventional radiology. Students can learn about the anatomy and practice their skills in a risk-free environment.
- Diagnostic Skills Development:
- Radiology Training: Medical imaging is integral to the education of future radiologists. Students learn to interpret X-rays, CT scans, MRIs, and other imaging modalities to diagnose various conditions.
- Pathology Integration: Understanding the correlation between medical imaging findings and pathology is vital for medical students. Integrating pathology education with imaging studies enhances diagnostic skills.
- Case-Based Learning:
- Clinical Cases: Incorporating real clinical cases, along with relevant imaging studies, into the curriculum allows students to apply their knowledge to practical scenarios. This fosters critical thinking and problem-solving skills.
- Interdisciplinary Collaboration:
- Team-Based Learning: Medical imaging promotes collaboration between different healthcare professionals, including radiologists, surgeons, and other specialists. Interdisciplinary case discussions and collaborative learning contribute to a more holistic understanding of patient care.
- Advanced Imaging Modalities:
- Nuclear Medicine and PET-CT: Introduction to advanced imaging modalities helps students understand their applications in diagnosing and monitoring diseases, particularly in fields like oncology.
- Functional Imaging: Learning about functional imaging techniques, such as functional MRI (fMRI) and positron emission tomography (PET), enhances students’ understanding of physiological processes.
- Continuing Medical Education (CME):
- Updates on Advances: Medical professionals, including radiologists, benefit from ongoing education to stay current with technological advances and new imaging modalities. CME activities often include the interpretation of challenging cases and discussions on emerging trends.
- Research and Innovation:
- Incorporating Research: Integrating medical imaging into research projects allows students to contribute to advancements in the field. This involvement fosters a research-oriented mindset and keeps students informed about the latest developments.
The integration of medical imaging into education is essential for producing well-rounded and competent healthcare professionals. It provides a bridge between theoretical knowledge and real-world applications, preparing students for the complexities of clinical practice. As technology continues to advance, the role of medical imaging in medical education is likely to evolve, offering even more sophisticated and immersive learning experiences.
Accelerated cancer research using AI
Artificial Intelligence (AI) is making significant contributions to the field of cancer research, accelerating the pace of discovery and improving the understanding and treatment of cancer. Here are several ways in which AI is being utilized to expedite cancer research:
- Medical Imaging Analysis:
- Radiomics: AI algorithms analyze medical images (such as CT scans, MRI, and pathology slides) to identify subtle patterns and biomarkers that may be indicative of cancer. This aids in early detection and precise diagnosis.
- Tumor Segmentation: AI helps automate the segmentation of tumors in medical images, facilitating the measurement of tumor size and assessment of treatment response.
- Genomic Data Analysis:
- Identifying Genetic Mutations: AI algorithms analyze vast amounts of genomic data to identify genetic mutations associated with specific cancers. This information is crucial for understanding the molecular basis of cancer and developing targeted therapies.
- Drug Response Prediction: AI models predict how individual tumors are likely to respond to different drugs based on their genomic profiles. This allows for more personalized and effective treatment strategies.
- Drug Discovery and Development:
- Drug Repurposing: AI analyzes existing drug databases to identify potential candidates for repurposing in cancer treatment. This approach can expedite the development of new therapies by leveraging existing drugs with known safety profiles.
- Virtual Screening: AI accelerates the drug discovery process by predicting the potential effectiveness of new compounds, streamlining the identification of promising drug candidates.
- Clinical Trial Matching:
- Patient Recruitment: AI helps match eligible patients to clinical trials based on their medical records and genomic profiles. This speeds up the recruitment process, ensuring that trials enroll suitable participants more efficiently.
- Trial Design Optimization: AI contributes to the design of more effective clinical trials by analyzing historical data and identifying optimal trial parameters.
- Data Integration and Knowledge Discovery:
- Integration of Multi-Omics Data: AI techniques integrate diverse datasets, including genomic, proteomic, and clinical data, to reveal complex relationships and identify novel biomarkers.
- Knowledge Graphs: AI is used to build knowledge graphs that connect disparate pieces of information, facilitating a better understanding of the relationships between genes, proteins, pathways, and diseases.
- Prediction and Risk Assessment:
- Cancer Risk Prediction: AI models analyze patient data to predict the risk of developing specific types of cancer. This can inform early screening strategies and preventive interventions.
- Prognostic Models: AI is employed to develop prognostic models that predict the likely course of a patient’s disease, helping guide treatment decisions.
- Natural Language Processing (NLP):
- Literature Mining: NLP techniques extract valuable information from the vast biomedical literature, helping researchers stay updated on the latest findings and accelerating the identification of relevant studies.
- Remote Monitoring and Personalized Care:
- Remote Patient Monitoring: AI enables continuous monitoring of cancer patients, providing real-time data on their condition and treatment responses. This contributes to personalized and proactive healthcare.
The integration of AI in cancer research not only accelerates the discovery of new insights but also enhances the precision and individualization of cancer diagnosis and treatment. Collaborations between data scientists, clinicians, and researchers are essential to harness the full potential of AI in the fight against cancer. Additionally, ensuring ethical considerations, data privacy, and regulatory compliance are crucial in the development and deployment of AI solutions in cancer research and healthcare.
AI medical devices
AI in medical devices refers to the integration of artificial intelligence technologies into various healthcare devices to enhance their capabilities, improve diagnostics, and provide more personalized and efficient patient care. Here are some examples of AI applications in medical devices:
- Diagnostic Imaging:
- AI-Assisted Radiology: AI algorithms can analyze medical images (X-rays, CT scans, MRIs) to assist radiologists in detecting abnormalities, such as tumors or fractures, and provide more accurate and timely diagnoses.
- Computer-Aided Diagnosis (CAD): CAD systems use AI to highlight potential areas of concern in medical images, aiding radiologists in their interpretation and decision-making.
- Cardiac Monitoring:
- AI ECG Analysis: AI algorithms can analyze electrocardiograms (ECGs) to detect irregularities in heart rhythms, providing early indications of conditions like arrhythmias or heart attacks.
- Wearable Devices: Wearable AI-enabled devices can continuously monitor heart activity, providing real-time data to healthcare professionals for early intervention.
- Glucose Monitoring:
- Continuous Glucose Monitoring (CGM): AI-based CGM systems help individuals with diabetes monitor their blood glucose levels more effectively. These devices can predict trends and provide alerts for potential hypoglycemic or hyperglycemic events.
- Drug Delivery Systems:
- Smart Infusion Pumps: AI-enhanced infusion pumps can adjust medication delivery based on real-time patient data, optimizing drug dosages and reducing the risk of medication errors.
- Closed-Loop Systems: AI-driven closed-loop systems for insulin pumps adjust insulin delivery based on glucose levels, offering a more automated and personalized approach to diabetes management.
- Personalized Medicine:
- Genomic Analysis Devices: AI is used to analyze genomic data, helping to identify genetic markers and variations that can guide personalized treatment plans.
- Point-of-Care Testing: AI-powered devices at the point of care can analyze patient samples, such as blood or saliva, providing rapid diagnostic information for personalized treatment decisions.
- Robot-Assisted Surgery:
- Robotic Surgical Systems: AI plays a role in robotic-assisted surgery, where algorithms help surgeons with image analysis, precision movements, and decision-making during procedures.
- Remote Patient Monitoring:
- AI Wearables: Wearable devices equipped with AI algorithms can monitor various health parameters, such as activity levels, heart rate, and sleep patterns. This data can be transmitted to healthcare providers for remote monitoring.
- Telehealth Devices: AI-enabled devices for telehealth applications can facilitate remote consultations and provide real-time health information to healthcare professionals.
- Cognitive Health Devices:
- Brain-Computer Interfaces: AI technologies can be integrated into devices that interface with the brain, enabling applications such as neurofeedback for cognitive health monitoring and rehabilitation.
- Wearable Brain Sensors: Wearable devices with AI algorithms can analyze brainwave patterns for early detection of conditions like epilepsy or neurodegenerative disorders.
As AI continues to advance, it is expected to play an increasingly prominent role in the development of innovative medical devices, contributing to improved diagnostics, personalized treatment plans, and enhanced patient outcomes. However, it’s important to consider regulatory compliance, data security, and ethical considerations when developing and deploying AI-based medical devices.